In the age of Artificial Intelligence (AI), data labeling often gets overshadowed by the marvels of machine learning algorithms. However, behind the scenes of every intelligent AI system lies a painstaking process – data labeling – where human expertise plays an irreplaceable role.
While automation advancements are making strides, the human touch in data labeling remains indispensable for ensuring accuracy, efficiency, and ethical considerations.
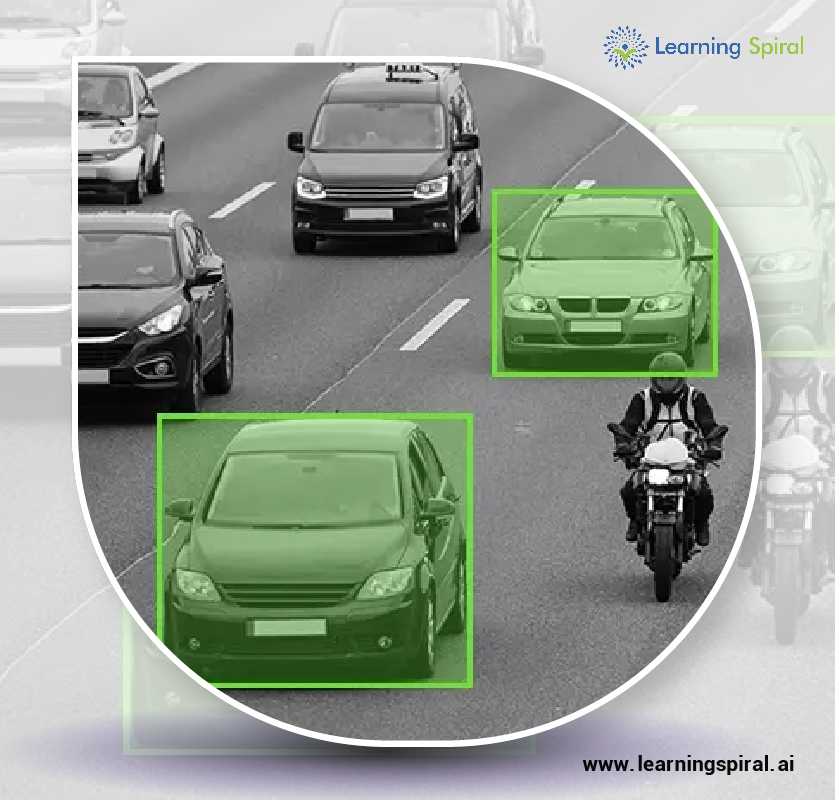
Understanding the Nuances:
The human brain is uniquely adept at interpreting context, understanding subtle cues, and recognizing complex patterns. This is crucial for data labeling tasks, where raw data needs to be meticulously categorized and annotated with precise information.
Images, for example, can contain a wealth of information beyond what simple algorithms can detect. A human labeler can distinguish between a harmless dog and a ferocious wolf, or identify the subtle difference between a smile and a smirk.
Maintaining Quality and Avoiding Bias:
Human expertise is vital for maintaining the quality of labeled data. Inconsistent labeling can lead to biased algorithms, hindering the performance of AI systems. Human labelers can identify and address inconsistencies, ensuring that annotations are accurate and consistent across the entire dataset.
Additionally, they can detect and mitigate biases that might be inadvertently introduced during the labeling process. For instance, facial recognition systems trained on data with limited ethnic diversity might exhibit racial bias.
Human experts can help identify and address such biases, promoting fairness and ethical AI development.
Beyond Automation:
While automation is making waves in data labeling, it cannot fully replace human expertise. Current AI-powered labeling tools are best suited for automating repetitive tasks with well-defined criteria. However, complex tasks involving judgment calls, nuanced interpretations, and understanding context still require human intervention.
Additionally, AI models themselves are trained on labeled data, so the quality of the training data directly impacts the performance of the AI. In this sense, human expertise becomes a vital part of the entire AI development lifecycle.
The Future of Human-AI Collaboration:
The future of data labeling lies in a collaborative approach between humans and AI. Automation can handle repetitive tasks, freeing up human labelers to focus on more complex aspects of the process.
AI tools can also assist with quality control measures, identifying inconsistencies and potential errors in labeling. This human-AI collaboration will lead to a more efficient and accurate data labeling process, ultimately paving the way for the development of more robust and reliable AI systems.
Beyond Technical Skills:
Human expertise in data labeling extends beyond technical skills. Domain knowledge is often crucial for tasks requiring specialized knowledge. For example, medical image annotation requires labelers with a deep understanding of human anatomy and medical terminology.
Similarly, legal document annotation needs individuals familiar with legal jargon and specific regulatory guidelines. By leveraging human expertise with domain-specific knowledge, data labeling can be tailored to specific industry needs, leading to improved AI applications within those fields.
Conclusion:
While AI automation is making significant strides, the human touch remains a critical element in data labeling. Humans contribute to ensuring the accuracy, efficiency, and ethical development of AI systems.
By fostering collaboration between humans and AI, we can unlock the full potential of data labeling and pave the way for a future where AI truly benefits society.