In the age of artificial intelligence aka AI, machines are constantly learning to understand and interact with the world around them. But when it comes to human language even the most sophisticated algorithms struggle to grasp the true meaning. The presence of multiple nuances and ambiguities makes this task difficult for advanced algorithms.
Here’s where linguistic annotation steps in, acting as a bridge between the complexities of language and the computational needs of AI models.
What is Linguistic Annotation?
Also known as corpus annotation, linguistic annotation is the process of enriching raw language data with additional layers of information. This data can be text, audio, or even video recordings.
Trained linguists or experts from the field work as annotators and add labels & tags that reveal the grammatical structure, semantic meaning, and even the emotions conveyed within the language.
Think of it like training wheels for AI models. By providing them with labeled examples, linguists help them learn to recognize patterns and make sense of the data.
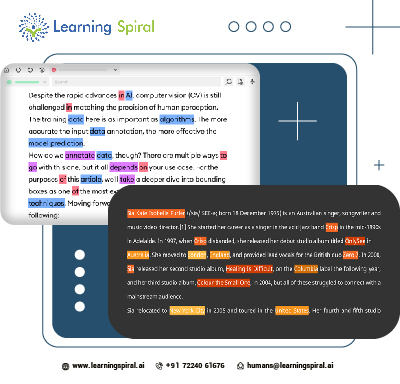
Why is Linguistic Annotation so Important?
Different types of linguistic annotation serve various purposes:
- Part-Of-Speech (POS) tagging: POS tagging refers to labeling each word in a sentence with its grammatical function (noun, verb, adjective, etc.). This helps AI models understand the sentence structure and identify relationships between words.
- Named Entity Recognition (NER): NER identifies and classifies named entities like people, organizations, locations, and dates within the text. This allows AI models to extract valuable information and use it for tasks like question answering or information retrieval.
- Sentiment Analysis: Labels the emotional tone of the text, classifying it as positive, negative, or neutral. This helps AI models understand the speaker’s or writer’s attitude and tailor their response accordingly.
Advantages of Linguistic Annotation
The benefits of linguistic annotation go beyond improving the accuracy and effectiveness of AI models. It also:
- Reduces ambiguity: Language is often open to multiple interpretations. Annotation helps clarify the intended meaning and reduce the chances of misinterpretations by AI models.
- Facilitates automation: By providing structure to unstructured data, annotation allows for the automation of various tasks, such as content moderation or generating tailored marketing messages.
- Improves data quality: The process of annotation itself can uncover errors and inconsistencies in the data, leading to a more reliable and valuable dataset for AI training.
However, creating high-quality linguistic annotations is no small feat. It requires highly trained personnel, specific guidelines, and robust annotation tools. That’s where experts like Learning Spiral comes in. These firms specialize in providing multiple services in the annotation sector to make the transformation smooth for businesses.
Despite the challenges, the impact of linguistic annotation on the development of AI models and natural language processing (NLP) is undeniable. This collaboration between linguists and AI researchers will pave the way for the creation of even more intelligent and sophisticated language-based technologies, shaping the future of human-computer interaction.