The rise of Artificial Intelligence (AI) has revolutionized various aspects of our lives. What most aren’t aware of is that to continue the advancement of AI models, the quality of data they are trained on needs to be updated. This is where Human-In-The-Loop (HITL) data labeling comes into play. It is a collaborative approach that integrates human expertise with machine intelligence to enable high-quality and accurate data labeling for machine learning.
The Power & Importance of Labeled Data:
Machine learning algorithms “learn” by analyzing vast amounts of data. This data needs to be labeled with relevant information – for example, identifying objects in an image or classifying the sentiment of a text snippet. High-quality labeled data sets are crucial for training robust and reliable AI models.
While automation has streamlined data labeling processes, it has its limitations. Complexities like ambiguity, context dependence, and nuances of language often pose challenges for purely automated labeling methods. Human understanding and judgment are essential for ensuring accurate and reliable labels.
The HITL Advantage:
HITL bridges the gap between automation and human expertise. Here’s how it enhances machine learning:
- Improved Accuracy: Human annotators can identify and address the shortcomings of automated systems, leading to more precise and consistent labeling.
- Handling Complexity: Human intelligence can tackle complex tasks like labeling ambiguous data, handling subjective interpretations, and understanding contextual nuances, leading to more robust AI models.
- Active Learning: HITL facilitates active learning, where AI models can identify areas of uncertainty and request additional human input to refine their understanding. This iterative process leads to continuous improvement in data quality and model performance.
- Domain Expertise: Incorporating domain-specific knowledge is crucial for specialized tasks like medical image analysis or legal document classification. Human experts with specialized knowledge can significantly enhance the quality of labelings in these domains.
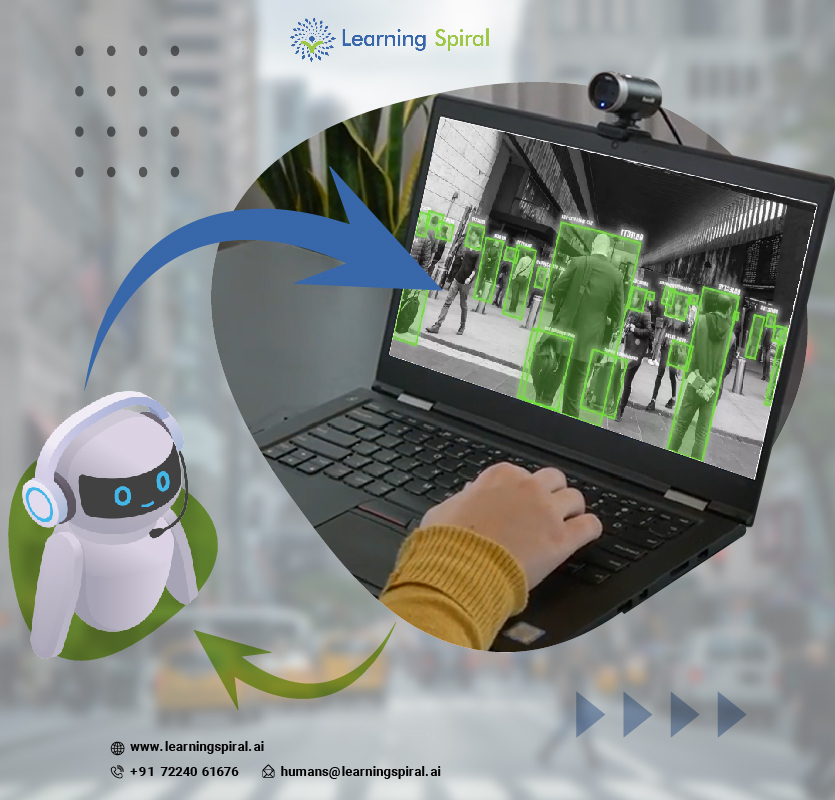
Intelligent Tools for Enhanced HITL:
Technology advancements are further empowering HITL workflows:
- labeling Tools: Advanced labeling platforms with intuitive interfaces and features like AI-assisted suggestions and quality control measures can streamline the labeling process for human annotators.
- Crowd-Sourcing: For specific tasks, leveraging a diverse pool of annotators through crowd-sourcing platforms can ensure varied perspectives and reduce bias.
- Data Augmentation: Artificial intelligence can be used to create synthetic training data, expanding datasets and improving model performance in specific scenarios.
The Future of HITL:
The future of HITL looks promising, with continuous advancements in AI and data labeling tools. Here are some key trends to watch:
- Focus on Efficiency: Integrating AI further will enhance automation capabilities, streamlining the HITL process and reducing manual burden on human annotators.
- Domain-Specific Specialization: Tailored labeling workflows and tools will cater to specific industry needs, ensuring high-quality labels for specialized AI applications.
- Democratization of AI Development: Enhanced HITL tools and platforms will empower smaller companies and researchers to participate in AI development by simplifying data labeling tasks.
Conclusion:
Human-in-the-loop data labeling plays a critical role in the success of machine learning projects. By combining human expertise with intelligent automation, this approach fosters the creation of accurate, reliable, and domain-specific labeled data.
As AI continues to evolve, HITL will remain a vital tool for unlocking the full potential of machine learning and shaping a brighter future for AI applications across various industries.