The rise of Artificial Intelligence aka AI has been fueled by one critical factor – data. But not just any data – high-quality, meticulously labeled data. This is where data labeling companies step in, playing a crucial role in training AI models to make accurate and unbiased decisions.
But how do these companies ensure the data they provide is both reliable and fair?
The Importance of Data Quality:
Imagine training an AI for facial recognition using poorly labeled images. It might misidentify people based on irrelevant factors like lighting or background clutter. This highlights the importance of data quality in labeling.
Here’s how companies ensure it:
- Clear and Comprehensive Guidelines: Detailed instructions with specific examples are provided to labelers to minimize ambiguity and ensure consistent labeling across the dataset.
- Multi-stage Labeling: Data may go through multiple rounds of labeling by different annotators, followed by a reconciliation process to identify and resolve discrepancies.
- Quality Control Measures: Statistical checks and human reviews are implemented to identify and rectify errors or inconsistencies in the annotations.
- Benchmarking and Validation: Labeled datasets are compared to established benchmarks or validated against pre-defined criteria to ensure they meet quality standards.
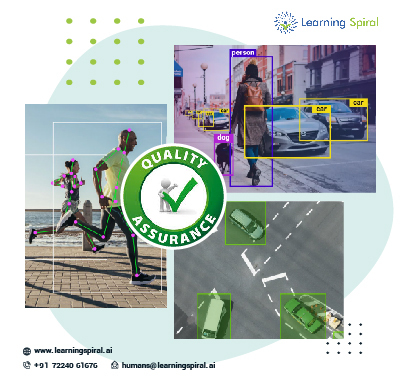
Combating Bias in Data Labeling:
Biases embedded in the data can lead to discriminatory AI models. Data labeling companies are actively addressing this challenge through:
- Diverse Labeling Workforce: Building a team of labelers with varied backgrounds and perspectives helps mitigate biases that might arise from a homogenous group.
- Blind Labeling: In certain scenarios, labelers might be unaware of the origin of the data (e.g., race, gender) to minimize the influence of unconscious bias.
- Algorithmic Bias Detection: Techniques like fairness audits can be used to identify potential biases within the labeled data and adjust the algorithms accordingly.
- Data Augmentation: Artificial creation of diverse data points can help balance datasets that might be skewed towards certain demographics.
Transparency and Collaboration:
Building trust in AI necessitates transparency in the data labeling process. Here’s how companies foster this:
- Clear Communication: Clients should be informed about the labeling methodology, quality control measures, and potential limitations of the data.
- Collaboration with Clients: Involving clients in the development of labeling guidelines and quality control checks can ensure the final dataset aligns with their specific needs.
- Focus on Explainability: Companies are exploring techniques to make AI models more transparent, allowing users to understand how the model arrived at a particular decision based on the labeled data.
The Evolving Landscape:
The field of data labeling is constantly evolving. Here are some trends to watch:
- Automation and AI Assistance: Machine learning is increasingly used to automate repetitive tasks in labeling and assist human annotators in making complex decisions.
- Active Learning: This technique identifies data points that hold the most value for model training, optimizing labeling efforts and improving efficiency.
- Focus on Explainable Labeling: Companies are developing tools that capture the rationale behind each label, providing valuable insights for interpreting and improving AI models.
Conclusion
Data labeling companies are the invisible hands behind the success of many AI applications. By ensuring data quality and fairness, they play a vital role in building trustworthy and ethical AI systems. As the field evolves, expect continuous innovation in labeling techniques and a growing emphasis on transparency and collaboration to pave the way for a more responsible future of AI.