The rise of artificial intelligence, popularly known as AI, hinges on one crucial element: data. But as data fuels innovation, concerns around data privacy and security in the world of data annotation for AI become paramount.
Sensitive information often flows through undetected while annotating data, raising ethical and legal challenges that demand careful consideration.
This article delves into the intricate relationship between data annotation, privacy, and security and explores strategies to find the right balance for responsible AI development.
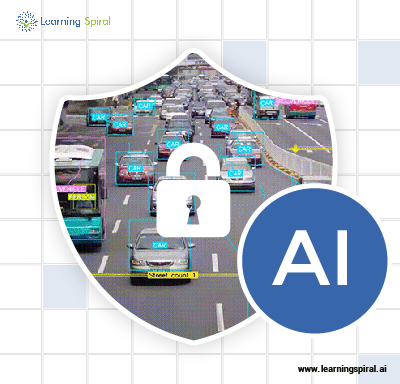
Understanding the Privacy Concerns:
Data annotation involves labeling and categorizing data, often by human annotators, to train AI models. This data can encompass:
- Personal information: Names, addresses, financial details, health records, and even facial recognition data can be present in various annotated datasets.
- Sensitive content: Images or videos depicting violence, medical conditions, or private gatherings pose privacy concerns.
- Proprietary information: Trade secrets, confidential documents, and product designs might be present in data used for industrial applications.
Privacy Risks and Ethical Issues:
Exposing such data during annotation carries inherent risks like:
- Data breaches: Unauthorized access to annotated datasets can lead to sensitive information leaks, causing harm to individuals and organizations.
- Misuse of data: Anonymized data can be re-identified, potentially breaching individual privacy and enabling profiling or discrimination.
- Algorithmic bias: Biased annotation practices can lead to discriminatory AI models, perpetuating societal inequalities.
Security Measures and Best Practices:
Among a multitude of practices, here are some security measures one can take to tackle this issue:
- Data anonymization and pseudonymization: Remove or mask identifiable information to minimize privacy risks.
- Secure platforms and tools: Utilize technology with robust encryption and access controls to protect data throughout the annotation process. Support and adopt innovative solutions that protect data privacy while enabling AI advancements.
- Privacy-aware training and protocols: Train annotators on data privacy principles and implement strict labeling guidelines to minimize bias and misuse. Promote public understanding of AI technology and the importance of data privacy and security.
- Regular security audits and assessments: Continuously evaluate and update security measures to address emerging threats.
- Transparency and disclosure: Inform data providers about how their data is used and anonymized, and provide avenues for redress if needed.
- Collaboration and communication: Establish open communication among data providers, annotators, AI developers, and regulators to build trust and understanding.
- Legal and regulatory compliance: Adhere to relevant data protection laws like GDPR (General Data Protection Regulation) and CCPA (Central Consumer Protection Authority) and follow ethical guidelines for AI development.
Conclusion:
Data privacy and security are not roadblocks to AI innovation; they are essential cornerstones for responsible development. By prioritizing these concerns, adopting robust security measures, and fostering collaboration, we can unlock AI’s potential while safeguarding individuals’ rights and privacy.
Remember, responsible AI requires functionality and ethical and secure foundations for a future where technology benefits all.