Medical imaging plays a crucial role in diagnosing and treating diseases. It encompasses X-rays, CT scans, MRIs, and more which are essential for life-saving. Artificial intelligence has the potential to revolutionize this sector by analyzing these images and assisting healthcare professionals. And data labeling helps make this process smoother and better.
Data labeling in medical imaging involves accurately identifying and annotating relevant features within an image. High-quality labeled data is the cornerstone for training effective AI models used in tasks like disease detection, treatment planning, and image segmentation.
Kinds of Data Labeling in Medical Area
There are several approaches to data labeling in medical imaging:
- In-house Labeling: Hospitals or research institutions can leverage their own medical staff, particularly radiologists with their expertise in image interpretation. This offers control over data quality but can be expensive and time-consuming.
- Crowdsourcing: Online platforms can be used to distribute labeling tasks to a wider pool of individuals, including medical students or trained annotators. This can be cost-effective but requires robust quality control measures to ensure accuracy.
- Automated Labeling Tools: Semi-automated or fully automated tools are emerging to assist with labeling tasks. These tools can pre-label images or highlight areas of interest, reducing human workload. However, they still rely on human expertise for verification and refinement.
Each approach has its advantages and disadvantages. Choosing the most suitable method depends on factors like project budget, data complexity, and required turnaround time.
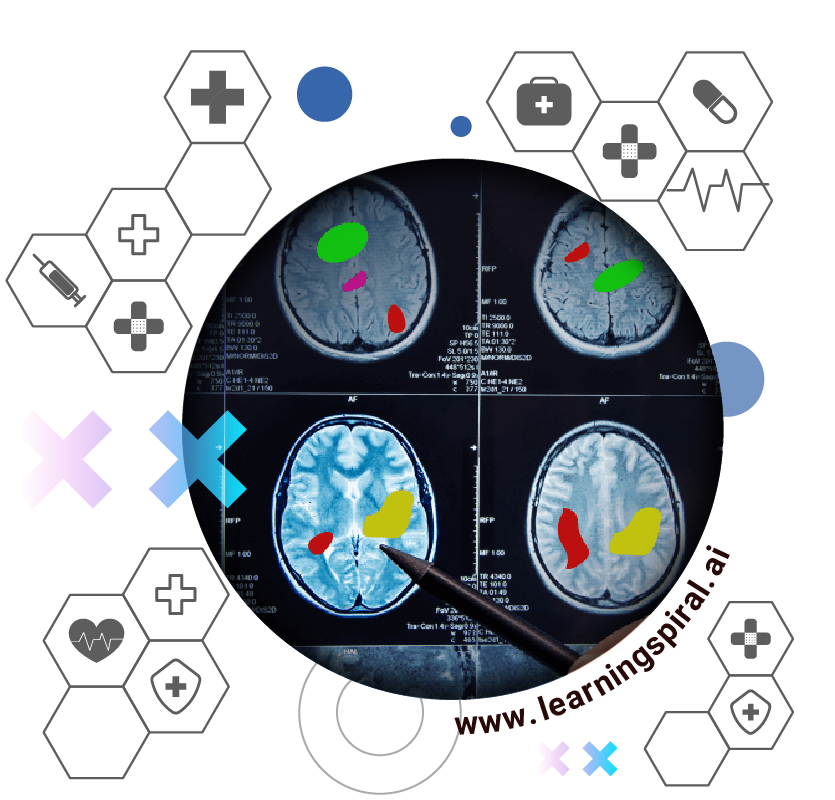
Challenges
However, data labeling for medical imaging is not without its pitfalls. Here are some key challenges to consider:
- Subjectivity and Variability: Medical images can be complex, and interpretation can vary between radiologists. This variability can lead to inconsistencies in labeling, impacting AI model performance.
- Data Bias: Biases can enter into labeled data if annotators unconsciously favor certain interpretations based on factors like patient demographics or previous diagnoses. This bias can be amplified by AI models trained on such data, leading to discriminatory outcomes.
- Cost and Time: Data labeling is a labor-intensive process, especially for complex tasks. Finding qualified personnel and ensuring quality control can be expensive and time-consuming.
- Data Privacy: Medical images contain sensitive patient information. Strict data privacy regulations need to be followed when collecting, storing, and labeling medical imaging data.
Places for Development
Despite these challenges, researchers are actively exploring solutions to improve data labeling practices in medical imaging. Here are some promising approaches:
- Standardization of Labeling Protocols: Establishing clear guidelines for labeling specific types of images can minimize variability and ensure consistency.
- Active Learning: This approach prioritizes labeling the most informative data points for the AI model, optimizing the labeling process and reducing human effort.
- Double Annotations and Adjudication: Having multiple experts label the same image and resolving discrepancies through expert review can improve labeling accuracy.
- Federated Learning: This technique allows training AI models on distributed datasets across multiple institutions without directly sharing patient data, addressing privacy concerns.
Conclusion
By acknowledging and addressing the challenges of data labeling, researchers and healthcare professionals can unlock the full potential of AI in medical imaging. High-quality, unbiased, and efficiently labeled data is essential for building robust and reliable AI models that can ultimately improve patient care and outcomes.