Data labeling is a crucial component of artificial intelligence and machine learning, laying the foundation for training algorithms to recognize patterns, make predictions, and drive intelligent decisions. Historically, data labeling was a labor-intensive process, requiring human annotators to meticulously tag data points across vast datasets manually. This traditional approach, while effective, often involved long hours, increased risk of human error, and significant costs.
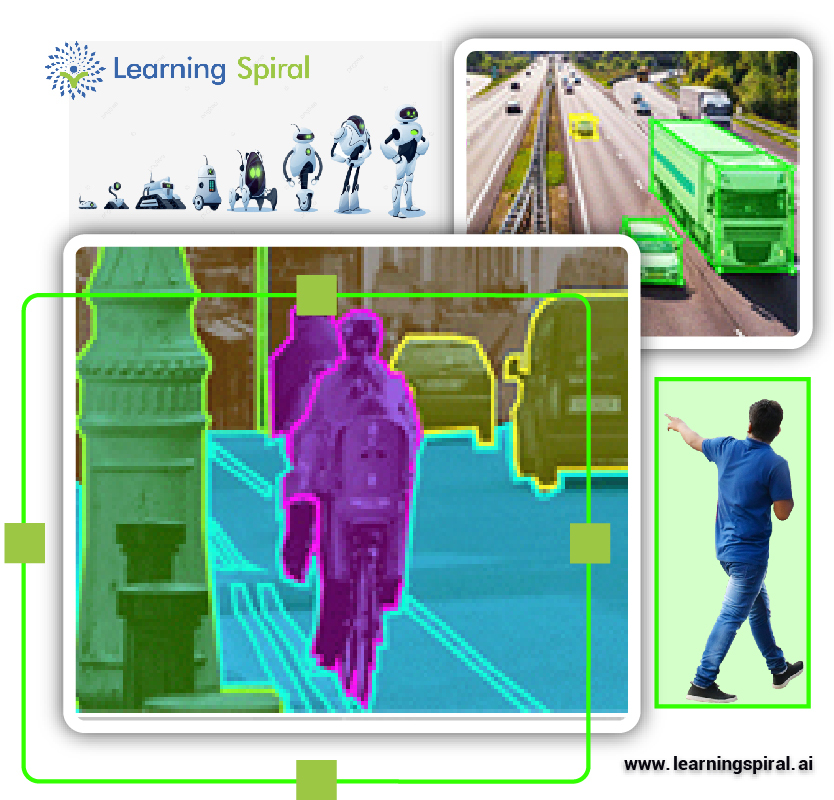
The landscape of data labeling has undergone a transformative shift with the advent of AI-driven processes. Today, machine learning models and advanced algorithms have revolutionized the way data labeling is approached. Automated tools now assist in the annotation process, significantly accelerating the speed and efficiency of data preparation.
AI-driven data labeling leverages sophisticated algorithms to perform tasks such as image recognition, text classification, and speech transcription with remarkable accuracy. These tools can handle massive volumes of data, learning from each iteration to improve performance over time. This transition from manual to automated processes not only enhances accuracy but also reduces the time and cost associated with data labeling.
Despite these advancements, human expertise remains vital in the data labeling process. AI tools benefit from human oversight to ensure the quality and relevance of annotations, especially in complex scenarios where nuanced understanding is required. By combining the efficiency of AI with the precision of human input, organizations can achieve a more balanced and effective data labeling approach.
At Learning Spiral AI, we embrace this evolution, integrating cutting-edge technologies with skilled human annotation to deliver high-quality data labeling solutions. As AI continues to advance, we are committed to staying at the forefront of this transformation, ensuring our clients benefit from the best of both worlds in data annotation.