The rise of Artificial Intelligence (AI) hinges on the quality and quantity of data used to train its algorithms. Data labeling, the meticulous process of tagging data with relevant information, plays a crucial role in this process.
However, with the increasing focus on data privacy, regulations are casting a long shadow on traditional data labeling practices. This article explores how these regulations are impacting the way data is labeled and the steps organizations can take to ensure compliance and ethical data practices.
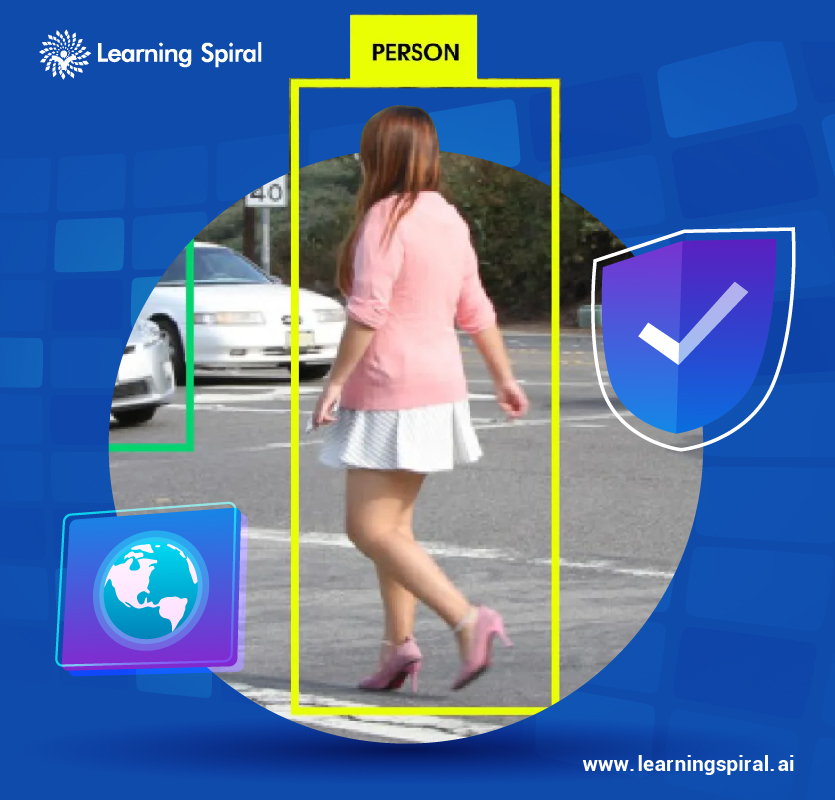
Privacy Concerns in Data Labeling
At the heart of the issue lies the potential for data labeling to expose sensitive information. Data used for AI training can encompass a wide range of sources, including text, images, and even audio recordings. If this data contains Personally Identifiable Information (PII) like names, addresses, or medical records, the labeling process could inadvertently reveal this sensitive data to labelers.
This raises concerns about individual privacy and potential misuse of personal information.
The Regulatory Landscape
Data privacy regulations like Digital Personal Data Protection Act (DPDPA) mandate transparency and control over how personal data is collected, used, and stored. These regulations require organizations to have a lawful basis for processing personal data and to implement appropriate security measures to protect it.
When it comes to data labeling, this translates to:
- Consent: Organizations need to obtain explicit consent from individuals before using their data for labeling purposes. This can be challenging, especially for data collected from public sources.
- Data Minimization: The amount of personal data used for labeling should be minimized. Techniques like anonymization and pseudonymization can help reduce the risk of exposing sensitive information.
- Security Measures: Robust security protocols are essential to safeguard data throughout the labeling process. This includes secure storage, access controls, and regular audits.
- Right to Erasure: Individuals have the right to request the deletion of their personal data. Organizations must have procedures in place to handle such requests efficiently.
Adapting Data Labeling Practices
The impact of data privacy regulations on data labeling practices is multi-faceted. Here’s how organizations are adapting:
- Synthetic Data: The use of synthetically generated data, which mimics real-world data but doesn’t contain any personal information, is gaining traction. This eliminates privacy concerns altogether.
- Federated Learning: This technique allows training models on decentralized datasets without physically moving the data. This minimizes the risk of exposing sensitive information.
- Privacy-Preserving Labeling Techniques: Techniques like differential privacy and homomorphic encryption can be employed to obfuscate sensitive information while still allowing for accurate labeling.
- Enhanced Security: Organizations are investing in robust security measures to protect data throughout the labeling workflow, including secure cloud storage and access controls.
- Transparency and Training: Labelers need to be aware of data privacy regulations and best practices for handling sensitive information. Providing clear guidelines and training is crucial.
The Road Ahead
Data privacy regulations are not roadblocks; they are catalysts for a more responsible approach to data labeling. By embracing these regulations, organizations can ensure compliance, build trust with users, and ultimately, develop more ethical and reliable AI models.
As AI continues to evolve, the interplay between data privacy and data labeling practices will necessitate continuous innovation and adaptation. By prioritizing privacy alongside efficiency, organizations can navigate this evolving landscape and unlock the full potential of AI.