Apart from education, artificial intelligence has played a major role in making facial recognition a possibility for the utmost class. Now, not only administrative officials or business personnel but ordinary people can also provide their properties with an extra level of privacy and security with facial recognition tools. In this regard, data annotation has a crucial standing.
Facial recognition systems rely on extensive datasets to accurately identify and authenticate individuals. Data annotation is crucial for training these models, involving the labeling of facial features, expressions, and other relevant details.
Importance of Data Annotation in Facial Recognition
Here’s why data annotation is essential in the context of facial recognition:
- Model Accuracy: Accuracy is the key to the right decision-making by any AI or machine learning model. Accurate annotations contribute to the training of facial recognition models, ensuring they can identify individuals reliably. Annotations may include facial landmarks, expressions, and other distinctive features crucial for precise recognition.
- Diversity and Inclusivity: People from all over the world contain a unique set of features, behaviors, and postures which make them stand out. This is where diversity and inclusivity come into play. Annotated datasets should encompass a diverse range of facial features, ethnicities, and genders to prevent biases in the facial recognition system. Inclusive data annotation helps in creating fair and unbiased models suitable for a wide range of demographics.
- Security Enhancement: With the rapidly advancing facial recognition tools and software, privacy is getting first priority, as it should. Annotated data assists in developing more secure facial recognition systems by incorporating features that distinguish between real faces and attempts to deceive the system using photos, masks, or other fraudulent means.
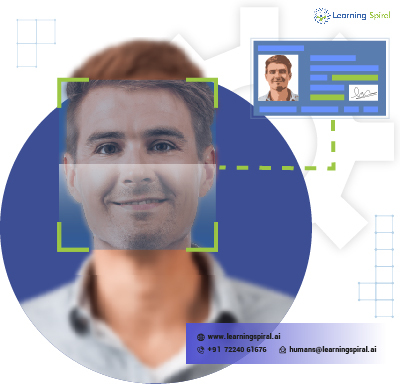
But, this advancement is not as easy as it seems. There are multiple challenges on the way.
Challenges in Data Annotation for Facial Recognition
- Informed Consent: Annotating facial data often involves using images of individuals. Obtaining informed consent from individuals for the use of their facial data in training datasets is crucial to respect privacy rights. Clear guidelines and transparency about data usage are essential.
- Data Anonymization: Facial images in datasets must be anonymized to prevent the identification of individuals. Anonymization techniques, such as blurring or using synthetic data, help protect privacy and comply with data protection regulations.
- Biases and Fairness: Bias in facial recognition systems, often stemming from biased training data, can lead to unfair and discriminatory outcomes. Rigorous annotation guidelines that promote diversity and fairness are necessary to mitigate bias in facial recognition models.
- Security of Annotated Data: Given the sensitive nature of facial data, ensuring the security of annotated datasets is crucial. Implementing robust encryption, access controls, and secure storage practices helps protect against unauthorized access or data breaches.
- Adversarial Attacks: Facial recognition systems can be vulnerable to adversarial attacks, where malicious actors attempt to manipulate or deceive the system. Annotating data with examples of potential attacks helps enhance the system’s robustness.
In this way, data annotation is essential for facial recognition. Balancing the potential benefits of facial recognition technology with privacy and security considerations requires careful attention to ethical practices in data annotation.